Introduction
Artificial intelligence (AI) has been making waves across various industries, bringing about a transformation that is nothing short of revolutionary. From healthcare to retail, the capabilities of AI have uncovered new avenues of efficiency and innovation. It’s only logical to expect that the world of finance would also be reshaped by this technological marvel.
Imagine AI as a sharp-suited finance guru, armed with algorithms and mathematical prowess, ready to take the financial world by storm. With its ability to process vast amounts of data, uncover hidden patterns, and make razor-sharp predictions, AI is gearing up to redefine finance as we know it. This isn’t a mere trend; it’s a paradigm shift that holds immense potential.
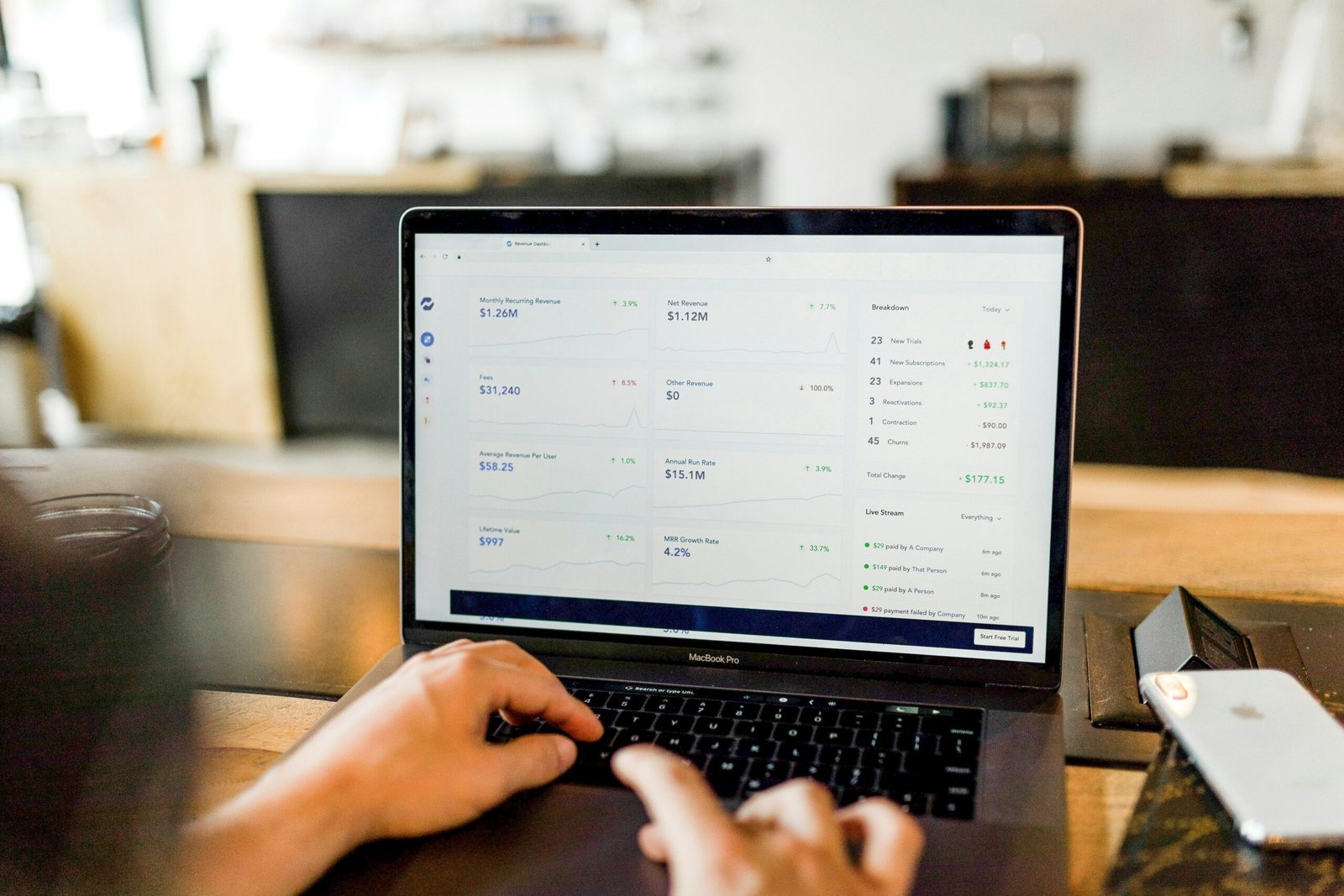
Within the realm of finance, two specific applications of AI stand out: predictive analytics and risk management. Predictive analytics, with its knack for forecasting market trends and customer behaviors, offers unprecedented insights, empowering financial institutions to make more informed decisions. On the other hand, risk management leverages AI’s data-crunching capabilities to identify potential risks and offer strategies to mitigate them efficiently. Together, these AI-driven applications are setting the stage for a more robust and resilient financial landscape.
As we delve deeper into how AI is revolutionizing finance, we’ll explore the nuances of these applications, examine their benefits, and look ahead to what the future might hold. Brace yourself as we embark on this riveting journey into the future of AI in finance, where algorithms and analytics are not just tools but pioneers of a new financial era.
What is Predictive Analytics in Finance?
Predictive analytics in finance refers to the use of statistical algorithms and machine learning techniques to analyze historical data and predict future financial trends or outcomes. At its core, predictive analytics combines vast amounts of historical financial data with advanced computational techniques to identify patterns and relationships that could inform future decisions. This process is fundamental in allowing financial institutions to make more informed and strategic decisions, thus minimizing risks and maximizing gains.
One of the primary applications of predictive analytics in finance is predicting stock market trends. By examining historical data on stock prices, trading volumes, and other relevant financial metrics, predictive models can forecast potential future movements in stock prices. These models might analyze patterns such as seasonal variations, economic indicators, and market sentiment to provide insights that traders can use to enhance their investment strategies.
Another essential application is in the realm of economic forecasting. Predictive analytics tools can help forecast economic shifts by analyzing historical economic data, including GDP growth rates, employment figures, inflation rates, and consumer spending. By identifying trends and relationships within this data, financial analysts and policymakers can better anticipate economic downturns or booms, thereby making more informed decisions around monetary policy and budget allocations.
Moreover, predictive analytics extends to risk management. By leveraging historical data on loan defaults, credit scores, and borrower behavior, financial institutions can assess the creditworthiness of potential borrowers more accurately. This enables them to set appropriate interest rates and loan conditions, thereby reducing the risk of default and improving overall financial stability.
In sum, predictive analytics in finance represents a fusion of big data and advanced analytical techniques, empowering financial entities to navigate the complex landscapes of financial markets and economic environments. Through accurate predictions and risk assessments, these tools contribute significantly to strategic planning and decision-making processes within the financial industry.
How Predictive Analytics is Changing Investment Strategies
The realm of investment strategies has been undergoing a transformative shift, driven by the emerging capabilities of predictive analytics and artificial intelligence. In today’s fast-paced financial markets, AI has become indispensable by uncovering trends and patterns that often evade even the most seasoned human analysts. Powered by vast datasets and advanced algorithms, AI-driven tools can process and analyze information at speeds unimaginable to humans, allowing for more informed and timely investment decisions.
Imagine a scenario where a human analyst, armed with years of experience and intricate knowledge of the stock market, competes against an AI-driven tool. While the analyst diligently scrutinizes stock volatility charts and economic indicators, the AI effortlessly crunches through terabytes of data in mere seconds, highlighting potential investment opportunities that might otherwise go unnoticed. The contrast is akin to a knight in armor jousting against a sleek, futuristic robot. While both have their strengths, the robot’s calibration driven by data precision might just edge out the human’s instinct and experience.
Traditional investment strategies often rely heavily on historical data, market sentiments, and economic indicators. Here’s a simplified comparison to illustrate the differences between traditional and AI-driven investment strategies:
Aspect | Traditional Investment Strategy | AI-Driven Investment Strategy |
---|---|---|
Data analysis speed | Relatively slow | Extremely fast |
Data handling capability | Limited to human capacity | Handles vast datasets efficiently |
Trend identification | Prone to human error | Highly accurate with continuous learning |
Adaptability | Limited and manual | Dynamic and automated |
As this comparison highlights, the integration of AI and predictive analytics into investment strategies offers a more dynamic, efficient, and precise approach. The ability of AI to predict stock volatility, optimize portfolios, and manage risks represents a paradigm shift for investors seeking to stay ahead in an ever-evolving financial landscape. While the human touch in finance remains invaluable, embracing AI-driven tools promises a future where human ingenuity and machine efficiency harmoniously coalesce for superior investment outcomes.
Risk Management: AI to the Rescue
The incorporation of Artificial Intelligence (AI) in the finance sector has revolutionized risk management strategies. Traditional risk assessment methods, while proficient in their own right, often fall short when it comes to processing and analyzing vast amounts of data in real-time. AI technologies, leveraging advanced machine learning algorithms and predictive analytics, can assess risks with heightened accuracy and efficiency. This enhanced precision stems from AI’s capability to evaluate extensive datasets and identify patterns that might elude human analysts.
To illustrate, let’s consider a hypothetical scenario within a large investment firm. The firm is evaluating a portfolio filled with various financial instruments. Traditionally, risk assessment would involve manual processes, extensive reports, and human intuition. However, with AI, the firm can deploy sophisticated algorithms to continuously monitor market conditions, historical data, and real-time trading information. By doing so, AI identifies subtle market signals indicating potential volatility in a particular asset class. This early detection allows the firm to reallocate assets and hedge appropriately, mitigating significant financial losses before they crystallize.
Advantages of AI in Risk Management
Several key advantages emerge when integrating AI into risk management frameworks:
- Real-time Data Analysis: AI systems can process and analyze incoming data instantaneously, offering up-to-the-minute risk assessments.
- Predictive Insights: By utilizing predictive analytics, AI anticipates potential risks before they materialize, allowing proactive measures.
- Enhanced Accuracy: AI reduces human error and biases by relying on data-driven insights and sophisticated algorithms.
- Scalability: AI can effortlessly scale its operations to analyze vast datasets, which is particularly beneficial for large financial institutions handling diverse portfolios.
- Cost Efficiency: Automating routine risk assessments can significantly reduce operational costs, freeing up resources for strategic decision-making.
AI’s role in risk management continues to evolve, offering an unprecedented level of precision and proactivity. As financial markets grow more complex, AI will be indispensable in safeguarding investments and ensuring the robustness of financial systems.
Case Studies: Success Stories of AI in Finance
AI technologies have made significant strides in the financial sector, particularly in predictive analytics and risk management. Key financial institutions like JPMorgan and Goldman Sachs have harnessed the power of AI to enhance their decision-making processes and mitigate risks. Below, we explore several case studies demonstrating the successful integration of AI in these areas.
JPMorgan Chase
JPMorgan Chase employs AI through its proprietary program, COiN (Contract Intelligence). COiN uses machine learning algorithms to analyze legal documents, significantly reducing the time required for complex contract review from 360,000 hours to just seconds. Furthermore, JPMorgan has integrated AI into its asset management services to predict market trends and optimize investment strategies.
Goldman Sachs
Goldman Sachs has developed AI technology for trading and risk management. Their predictive analytics tools forecast market movements and execute trades at optimal times, enhancing profitability while reducing exposure to risk. Goldman Sachs also uses AI to evaluate credit risk, ensuring more accurate assessments of borrower reliability.
Barclays
Barclays deploys AI to improve customer service and fraud detection. Their AI-powered chatbots handle an array of customer queries, streamlining client interactions and reducing operational costs. Additionally, Barclays’ AI-driven systems constantly monitor financial activities for anomalous patterns, thereby preventing potential fraud in real-time.
Morgan Stanley
Morgan Stanley leverages AI to augment the capabilities of its financial advisors. The company’s AI platform, known as Next Best Action, provides advisors with data-driven insights and personalized recommendations for clients, leading to more informed investment decisions and enhanced client satisfaction.
The table below summarizes the key takeaways from these case studies:
Company | AI Initiative | Key Results |
---|---|---|
JPMorgan Chase | COiN (Contract Intelligence) | Drastically reduced contract review time |
Goldman Sachs | Predictive Analytics for Trading and Risk Management | Enhanced trading profitability and reduced risk |
Barclays | AI Chatbots and Fraud Detection | Improved customer service and real-time fraud prevention |
Morgan Stanley | Next Best Action Platform | Data-driven insights for financial advisors |
These case studies underscore the transformative potential of AI in finance, illustrating how predictive analytics and robust risk management frameworks can revolutionize business operations and provide a competitive edge.
Challenges and Ethical Considerations
In the grand odyssey of integrating AI into finance, it’s not all algorithms and smooth sailing. While the potential benefits are enormous, several hurdles stand in the way, requiring a balanced, thoughtful approach. Let’s dive into some of these challenges and ethical considerations, reminding ourselves that embracing AI isn’t a walk in the park.
First, data privacy remains a significant concern. With banks and financial institutions amassing colossal quantities of personal data, the risk of breaches is higher than ever. Protecting sensitive information from unauthorized access or cyberattacks is paramount. However, with great data comes great responsibility, and ensuring that guidelines like GDPR are rigorously followed is non-negotiable.
Next up, algorithmic bias is another elephant in the digital room. Algorithms, while designed to be objective, can inadvertently reflect the biases of their creators or the data they trained on. This bias can lead to unfair lending practices, discriminatory insurance premiums, and much more. Ensuring that AI systems are transparent and regularly audited for fairness is crucial to maintaining trust and equity in financial services.
Let’s not overlook job displacement. The rise of AI has many professionals wondering if their jobs are next on the chopping block. While it’s true that automation can streamline operations and reduce the need for certain roles, it also paves the way for new opportunities in tech oversight, AI maintenance, and ethical compliance. In essence, while some jobs may evolve or vanish, new positions will emerge, requiring a skillful workforce willing to adapt.
To succinctly summarize, here are the key challenges and ethical considerations that need to be addressed in the realm of AI in finance:
- Data privacy protection and adherence to regulations
- Mitigation of algorithmic bias and ensuring fairness
- Navigating the potential for job displacement and fostering new opportunities
So, while the journey to a fully AI-integrated financial world is thrilling, it necessitates a cautious trek through these challenges. Fostering an AI ecosystem that values ethics and accountability is not just essential but critical for the future of finance.
The Future Landscape: What to Expect
The landscape of artificial intelligence (AI) in finance is poised for transformative changes over the next decade. One of the emerging trends is the integration of blockchain technology with AI. This fusion is anticipated to revolutionize data security, ensuring enhanced transparency and reducing the risks associated with fraud. Blockchain’s decentralized nature combined with AI’s analytic power can create a robust framework for real-time financial auditing and transaction verification. This trend could be effectively illustrated through an infographic showing the synergy between blockchain and AI in enhancing security protocols.
Additionally, there is significant potential for AI to enable more personalized financial services. Leveraging vast amounts of consumer data, AI algorithms can offer tailored financial advice, customized investment opportunities, and personalized customer service experiences. Imagine an AI-driven financial advisor that not only understands your current financial health but also anticipates your future needs based on detailed analytics. This personalized approach can enhance customer satisfaction and loyalty, a trend that can be visualized through a chart depicting increasing user engagement and satisfaction metrics over time due to personalized services.
Looking ahead, the adoption of AI across various financial domains is set to accelerate. Predictive analytics will play a pivotal role in risk assessment, enabling financial institutions to foresee market trends and potential threats. This will be crucial in navigating economic uncertainties and ensuring financial stability. A timeline chart could effectively capture the progression of AI-driven predictive analytics from current applications to future advancements.
The next 5 to 10 years will likely witness AI becoming an integral part of regulatory compliance, assisting in the automatic monitoring and reporting of compliance-related activities. This will not only streamline processes but also minimize human error. An infographic illustrating the workflow from data collection to compliance reporting powered by AI could provide a clear visual representation of this trend.
Overall, the future of AI in finance holds immense promise. As we move forward, the industry’s reliance on AI for predictive analytics and risk management will deepen, shaping a more secure, personalized, and efficient financial ecosystem.
Conclusion and Key Takeaways
As we conclude our exploration of the future of AI in finance, it is evident that predictive analytics and risk management are being fundamentally transformed by artificial intelligence. Today, AI-driven models are not only enhancing the accuracy of financial forecasts but are also equipping institutions with robust tools to mitigate risks. This evolution ushers in a new era where decision-making is data-driven, informed, and agile.
Predictive analytics stands at the forefront, empowering financial entities to sift through vast troves of data to unveil patterns and forecast trends with unprecedented precision. Meanwhile, AI’s role in risk management is becoming indispensable, as sophisticated algorithms offer real-time insights and preemptive measures against potential threats. These advancements not only improve operational efficiency but also bolster the overall stability of financial systems.
Yet, we are merely at the cusp of what AI can achieve in finance. Future developments promise enhanced personalization of financial services, greater fraud detection capabilities, and a seamless integration of AI into everyday operations. Ensuring that one stays abreast of these innovations is crucial for both financial professionals and organizations aiming to harness the full potential of AI technologies.
We encourage our readers to actively explore AI solutions tailored to their specific financial needs. Whether you are a financial institution or an individual investor, embracing AI advancements can significantly elevate your strategic planning and operational performance. Additionally, staying informed about upcoming technologies is imperative as the landscape of finance continues to evolve at a rapid pace.
In summary, the integration of AI into predictive analytics and risk management is not just enhancing current practices but is set to revolutionize the financial industry as a whole. The synergy between AI and finance promises a future where predictive insights and risk mitigations are more accurate, timely, and impactful than ever before.
FAQs
What is the role of AI in stock market prediction?
AI plays a significant role in stock market prediction by leveraging vast amounts of financial data and complex algorithms to identify patterns and trends. Machine learning models can analyze historical data, news articles, and financial reports to make informed predictions on market movements. The use of predictive analytics in AI allows for more accurate forecast models, helping investors make data-driven decisions.
How does AI improve risk management in banks?
AI enhances risk management in banks by automating the analysis of large volumes of data to detect anomalies and potential risks. Machine learning algorithms can assess credit scores, detect fraudulent activities, and predict market risks more efficiently than traditional methods. By continuously monitoring and analyzing data, AI systems offer real-time insights, allowing banks to proactively manage and mitigate risks.
What are the ethical concerns with AI in finance?
The integration of AI in finance raises several ethical concerns, such as data privacy, algorithmic bias, and transparency. The use of personal data for AI-driven decisions necessitates robust data protection measures. Additionally, ensuring that AI systems operate without biases is critical to prevent unfair treatment of individuals. Transparency in AI decision-making processes is essential to maintain trust and accountability.
Can AI replace human financial analysts?
While AI can significantly enhance the capabilities of financial analysts by providing data-driven insights and automating routine tasks, it is unlikely to replace human analysts entirely. Human expertise and judgment remain crucial for interpreting complex financial scenarios and making strategic decisions. AI serves as an invaluable tool that complements and augments human analysts’ skills.
What are the future prospects of AI in finance?
The future of AI in finance is promising, with continuous advancements in technology leading to more sophisticated predictive analytics and risk management solutions. The integration of AI is expected to drive innovation, improve decision-making processes, and enhance overall financial stability. As AI technologies evolve, their applications in finance are likely to become more widespread, offering increased efficiency and accuracy in financial operations.